4 Ways to Leverage Machine Learning to Predict Customer Churn in Subscription Services
In the rapidly evolving field of subscription services, predicting customer churn is critical. We’ve gathered insights from top industry professionals, including a Staff Machine Learning Engineer and a CEO & Founder, to bring you four specific tips and techniques. From tracking usage metrics and support tickets to engaging at-risk customers with predictive analytics, discover how machine learning can transform your customer retention strategy.
Want to get quoted in MarketerInterview.com content just like this? Apply to become a contributor today!
Contents
Track Usage Metrics and Support Tickets
It is possible to predict customer churn with high accuracy using machine learning (ML). The level of accuracy depends on the granularity of the dataset and the features used to build the ML model. It is important to track features like usage metrics, number of support tickets raised in a given timeframe, etc., to classify customer churn as “Likely” or “Unlikely.” These features directly indicate how the customer has been using your product and how happy or unhappy they are with it.
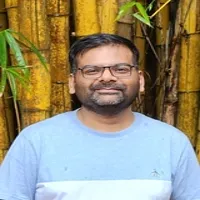
Pushkar Garg, Staff Machine Learning Engineer, Clari
Predict Churn With Proprietary Deep-Learning
This one particular tip is to predict negative dynamics in app use as early as possible.
At Lemon AI, we developed our own proprietary machine-learning architecture that predicts customer churn based on their in-app behavior. Based on a wide range of app-use patterns, our deep-learning models can, for instance, predict that a particular user is likely to stop using the app in a month.
To do so, the model takes into account multiple factors such as time spent or actions performed in-app, as well as the recurrence of the identified patterns over a certain time frame. Then, it can forecast a decrease in this particular user’s LTV and send a “red flag” to the Customer Service Team. It becomes a signal that they need to approach the user personally (e.g., via a dedicated manager or special customized offers), suggest new features, or promptly undertake other actions to prevent that churn.
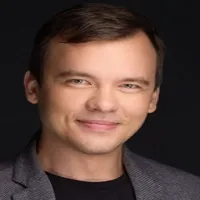
Andrew Bluemental, CEO & Co-Founder, Lemon AI
Combine Random Forest and Gradient Boosting
To effectively leverage machine learning for predicting customer churn in subscription services, focus on building a robust predictive model using historical customer data. One specific technique is to utilize a combination of Random Forest and Gradient Boosting algorithms. Start by gathering comprehensive data on customer interactions, including usage patterns, payment history, customer support interactions, and demographic information. Preprocess the data to handle missing values, normalize features, and encode categorical variables.
Train the Random Forest algorithm to identify key features influencing churn, as it excels at handling large datasets and capturing feature importance. Then, apply Gradient Boosting to refine the model by iteratively correcting errors made by the Random Forest, thus improving prediction accuracy. Evaluate the model using metrics like precision, recall, and the F1 score to ensure reliability. By continuously monitoring and updating the model with new data, you can proactively identify at-risk customers and implement targeted retention strategies, ultimately reducing churn rates.
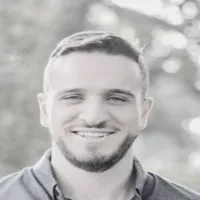
Omer Lewinsohn, General Manager, Marketing Expert, Management.org
Engage At-Risk Customers With Predictive Analytics
Use machine learning models to analyze customer behavior patterns and identify early signs of churn. Implementing predictive analytics with tools like Python’s Scikit-learn has allowed us to proactively engage at-risk customers with targeted retention campaigns. This approach reduced our churn rate by 20%, demonstrating the power of data-driven strategies in customer retention.
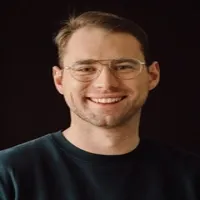
Alex Stasiak, CEO & Founder, Startup House
Want to get quoted in MarketerInterview.com content just like this? Apply to become a contributor today!