8 Ways to Use Predictive Analytics for Customer Behavior Forecasting
Diving into the world of predictive analytics for customer behavior forecasting, we’ve gathered insights from eight seasoned professionals including CEOs and Founders. They share their wisdom from utilizing AI-enabled data lakes to combining advanced segmentation with machine learning, offering a comprehensive guide on enhancing your forecasting techniques.
Want to get quoted in MarketerInterview.com content just like this? Apply to become a contributor today!
Contents
- 1 Utilize AI-Enabled Data Lakes
- 2 Leverage Historical Purchase Data
- 3 Implement Dynamic Micro-Segmentation
- 4 Analyze Data with Machine Learning Models
- 5 Anticipate Peak Demand with Real-Time Data
- 6 Build Predictive Models from Key Indicators
- 7 Predict Purchasing Patterns with CRM Data
- 8 Combine Advanced Segmentation with Machine Learning
Utilize AI-Enabled Data Lakes
At Innerverse, we heavily rely on our AI-enabled data lake and advanced language models like GPT-4 to perform predictive analytics for customer behavior forecasting. One specific technique we use is to combine data from multiple sources, such as product usage metrics, customer support interactions, social media sentiment, and CRM data, to create a holistic view of each user’s journey.
By feeding this rich, multi-dimensional data into our LLM, we can uncover patterns and correlations that might not be apparent when looking at individual data sources in isolation. For example, we might discover that users who engage with a particular feature within our AI-powered simulations and also express positive sentiment on social media are more likely to upgrade to a premium subscription.
The key to successful predictive analytics for customer behavior forecasting is to continuously refine and update your output based on new data and feedback. By leveraging an AI-enabled data lake and advanced language models, you can create a virtuous cycle of data collection, analysis, and action that helps you deliver the best experience for customers while keeping operating costs low.
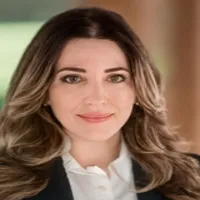
Lindsay Richman, Co-Founder, Innerverse
Leverage Historical Purchase Data
If you’re getting into predictive analytics for customer behavior, you’re going to need to start looking into properly using machine-learning algorithms on whatever historical purchase data you have available or can buy, specifically to identify patterns and trends.
You’re going to be looking to leverage variables including, but not limited to, purchase frequency, average order value, time between purchases, and product preferences to build a predictive model for future buying behavior.
If you can incorporate demographic and psychographic data on behavioral indicators, all the better. Alternatively, you can always take the easy way out and buy a Nielsen study if you have the budget.
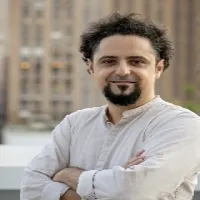
Dragos Badea, CEO, Yarooms
Implement Dynamic Micro-Segmentation
I worked at Amazon for four years as a software engineer on the Amazon Fulfillment Technology team, which powered all the fulfillment centers globally.
One effective technique for using predictive analytics to forecast customer behavior is to implement dynamic micro-segmentation. This involves continuously updating customer segments based on real-time data changes, allowing for highly personalized marketing actions that can significantly improve customer retention and sales.
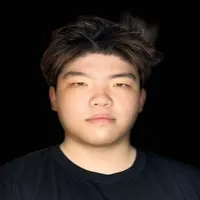
Peter Wang, Founder, Exploding Insights
Analyze Data with Machine Learning Models
Use machine learning models to analyze historical customer data and predict future behaviors. You can use machine learning algorithms to identify crucial patterns and trends in your customer data to help you make more accurate forecasts about customer actions, needs, and preferences.
Start by gathering comprehensive historical data on your customer interactions, including browsing behavior, purchase history, email responses, and social media engagement. Clean the data to ensure it is accurate and consistent before subjecting it to feature engineering to identify and create relevant features to help your machine learning model understand customer behavior.
Choose an appropriate machine learning model for your predictive analytics and train it on a subset of your data. Make sure you validate its performance using a separate data subset. Once the model is fully trained and validated, deploy it to start making valuable predictions on new customer data.
Although our business model mainly focuses on email deliverability, our in-house AI technology is trained to provide insights into customer engagement and preferences, which we use to train our predictive analytics model.
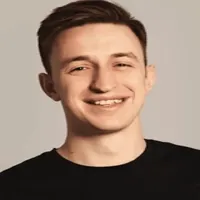
Vladislav Podolyako, Founder and CEO, Folderly
Anticipate Peak Demand with Real-Time Data
One effective tip for using predictive analytics for customer behavior forecasting is leveraging real-time data to anticipate peak service demand times. At TRAX Analytics, we’ve implemented this technique extensively. For example, in airport operations, analyzing historical flight data combined with real-time passenger flow enables us to predict high-traffic periods. This allows us to allocate resources such as custodial staff more efficiently, ensuring that high-use areas like restrooms are adequately maintained.
A concrete example: By comparing passenger traffic patterns from previous years with current data, we identified that Saturdays between 10 AM and 1 PM were peak times for restroom usage at a major airport. Based on this prediction, we increased staffing levels during these hours, resulting in a 25% reduction in customer complaints related to restroom cleanliness.
This same approach can be adapted for other industries. Analyzing customer touchpoints—whether through website traffic, purchase history, or foot traffic in retail stores—allows companies to anticipate high demand periods and adjust staffing or inventory levels accordingly. It’s a proactive strategy that leads to higher customer satisfaction and operational efficiency.
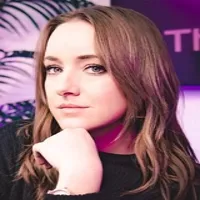
Tracy Davis, Founder and CEO, TRAX Analytics, LLC.
Build Predictive Models from Key Indicators
Utilize your historical data to forecast future customer behavior accurately. What you need to do is analyze patterns and trends from past interactions. For example, track which online searches commonly lead to conversions or which cases bring repeat clients. Identify these key indicators and use them to build predictive models.
Once your models are set, apply them to predict future behavior. Anticipate which clients are likely to need legal assistance again and tailor your marketing efforts to these high-value targets. This focused approach not only boosts your efficiency but also increases client satisfaction by addressing their needs proactively.
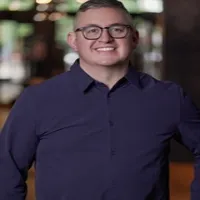
Casey Meraz, Owner and Digital Marketing Expert, Casey Meraz
Predict Purchasing Patterns with CRM Data
One specific technique I’ve found effective for using predictive analytics in customer behavior forecasting is leveraging historical customer interaction data to develop machine-learning models that predict future purchasing patterns. For instance, during my entrepreneurial stint with PacketBase, I used predictive analytics to track the behavior of high-value customers by analyzing their previous purchasing patterns, frequency of interactions, and response to past marketing campaigns.
A concrete example from my experience at PacketBase: Our data indicated that customers who engaged with our blog posts and web content on tech updates were more likely to upgrade their service packages within three months. By feeding this data into our predictive models, we were able to anticipate these upgrades and tailor our marketing efforts to this specific segment. This proactive approach resulted in a 20% increase in service upgrades, clearly demonstrating the power of predictive analytics in customer behavior forecasting.
In consultancy work with startups, I used similar predictive techniques to enhance direct-to-consumer (D2C) marketing strategies. By leveraging CRM data, I could identify behavioral triggers that indicated a higher likelihood of purchase. For example, for a SaaS product, users who completed a specific sequence of onboarding steps within the first seven days were 30% more likely to become paying customers. Tailoring follow-up emails and promotions based on these insights not only improved user engagement but also significantly boosted conversion rates.
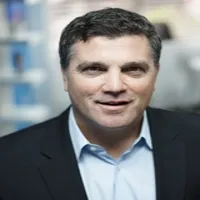
Gary Gilkison, Principal Analyst, Riverbase Cloud
Combine Advanced Segmentation with Machine Learning
Combining advanced segmentation with machine-learning models significantly enhances the precision of predictive analytics in customer behavior forecasting, providing deeper insights into customer actions.
Technique Overview
1. Data Collection and Preparation:
– Collect comprehensive customer data, including transactions, interactions, social media activities, and demographics.
– Ensure data quality through cleaning and normalization.
2. Customer Segmentation:
– Use clustering algorithms like K-means or DBSCAN to segment customers based on behavior, preferences, and value.
– Include static attributes (age, location) and dynamic behaviors (purchase frequency, product preferences).
3. Feature Engineering:
– Create features capturing customer lifecycle stages, engagement levels, and potential value, such as RFM scores, churn indicators, and product affinity scores.
– Use domain-specific knowledge to derive features predicting future behaviors like seasonality effects.
4. Predictive Modeling:
– Apply models like Gradient Boosting Machines (GBM), Random Forests, or Neural Networks to predict behaviors such as purchase likelihood or churn probability.
– Train models on segmented data to improve accuracy.
5. Model Evaluation and Refinement:
– Use metrics like AUC-ROC and Mean Absolute Error (MAE) to evaluate performance.
– Refine models through cross-validation and hyperparameter tuning.
Benefits
– Personalized Insights: Tailor marketing strategies and customer service by understanding different segments’ needs and behaviors.
– Enhanced Predictive Accuracy: Segmented models capture nuances, leading to more accurate forecasts.
– Proactive Interventions: Identify at-risk segments for timely interventions to reduce churn and focus on high-value segments to maximize retention.
An e-commerce company can cluster customers into segments like “Frequent Buyers,” “Seasonal Shoppers,” and “Discount Seekers.” Specialized models for each segment can predict behaviors such as purchase frequency and product preferences, allowing personalized marketing campaigns that increase engagement and conversion rates.
Advanced segmentation combined with predictive modeling is key for accurate customer behavior forecasting. It enables businesses to fully utilize their data, offering actionable insights that drive strategic decisions and enhance customer experiences.
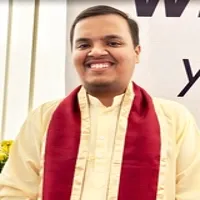
Ashish Bhanushali, Associate Business Analyst, Wappnet Systems Pvt Ltd
Want to get quoted in MarketerInterview.com content just like this? Apply to become a contributor today!